In a world where bad actors are focused on building sophisticated AI capable of side-stepping traditional Cybersecurity platforms, it has become critically important to onboard tools that work in real-time, are exceedingly precise, and can detect an incident before it happens.
Yesterday’s solutions are no match for today’s threats. MixMode believes the answer lies in self-supervised AI that can leapfrog the abilities of adversaries to protect enterprise assets before damage is done.
What is self-supervised AI and why is it superior to supervised AI?
Respected AI researcher Yann LeCunn recently stated that “the next revolution in AI will not be supervised;” whereas almost all legacy Cybersecurity providers utilize supervised AI or off-the-shelf machine learning as the backbone of their artificial intelligence. LeCunn states that self-supervised learning, which in contrast to supervised learning does not require human labeling and results in a constantly evolving forecast, is “the future of AI.”
What does LeCunn mean by self-supervised AI? In simple terms, self-supervised AI uses algorithms to identify patterns among data that has not been classified or labeled by humans. This is in direct contrast to legacy Cybersecurity approaches, which rely on supervised AI built on labeled training data and human-written rules; with these legacy approaches, events that are not contemplated in training data or encompassed in the rule set go largely unnoticed.
Third Wave AI, as defined by DARPA, is AI that has contextual and explanatory models that are self-learning and self-supervised. Third Wave AI is never dependent on rules-based systems like SIEM in the Cybersecurity world. Third Wave self-supervised AI does not require labeling, or training data, or even human operator involvement, since it develops an evolving forecast of what’s expected based on enterprise data flow.
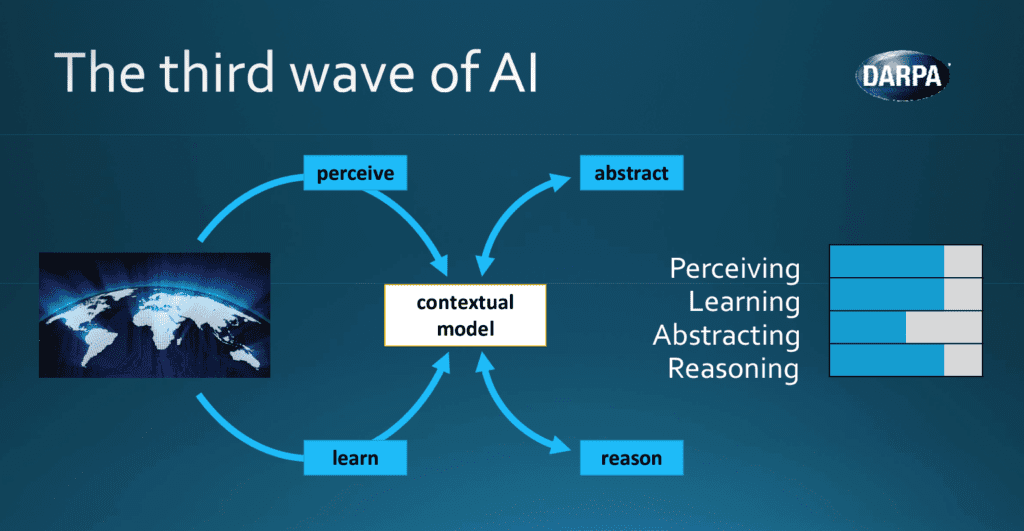
A DARPA Perspective on Artificial Intelligence
A Third Wave, self-supervised approach makes an enormous day-to-day difference over legacy approaches when it comes to two common pain points faced by modern SOCs:
- Managing huge numbers of false positive alerts
- Detecting Novel attacks, including Zero-day and insider threats
Each of these issues can wreak havoc on the effectiveness of SOC teams. False positives take up analyst time that could be better spent on shoring up system vulnerabilities and other security-related tasks. They also cloud the analysts’ view of the truly important alerts that may represent an imminent threat. In addition, the Ponemon Institute notes that Novel Attacks cost the global community around $2.5 trillion annually and now make up 80% of successful attacks on organization endpoints.
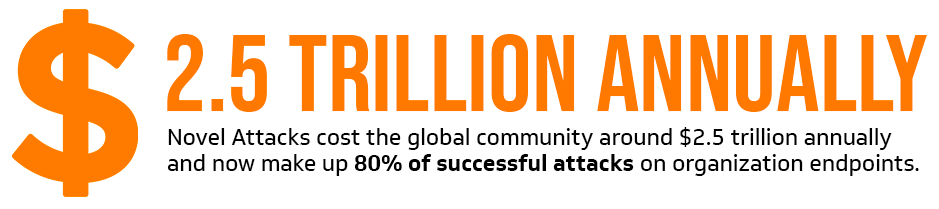
Rules-based, Second Wave approaches fall short
Bad actors today design their exploits to bypass legacy systems that rely on rules-based detection platforms. These legacy platforms typically utilize Second Wave regression or Bayesian-based machine-learning and are not able to detect novel anomalies, since the number of rules required would be infinite, and no IOC or training data exists for detecting a novel attack.
In addition to being ineffective at detecting novel attacks, these legacy approaches are dangerously exploitable, for several key reasons:
- Inherent biases and blindspots created by human input (e.g. man-made rules, finite training data, subjective alert suppression)
- Statistical limitations
- Historical training data requirements that necessitate unwieldy, expensive data stores
- An inability to contextually understand usage at different points
- An inability to adapt to new devices as they are added to networks
To detect novel threats, a self-supervised AI is required to automatically learn an environment with no requirement for training data or rules. Absent human training or tuning, bias and blindspots are eliminated in favor of the predictive forecast of normal behavior dictated by the data flows themselves. Advanced AI platforms should be able to ingest billions of records per day, like Flow Logs and CloudTrail data, to isolate only the high priority alerts of anomalous behavior, while providing visibility across all types of data streams.
As a self-supervised platform’s processing layer compares real-time data flows with the evolving forecast, it identifies anomalies based on discrepancies outside expected behavior. Risk levels and predictions should be provided to the user, along with all the underlying context data, available with a single click.
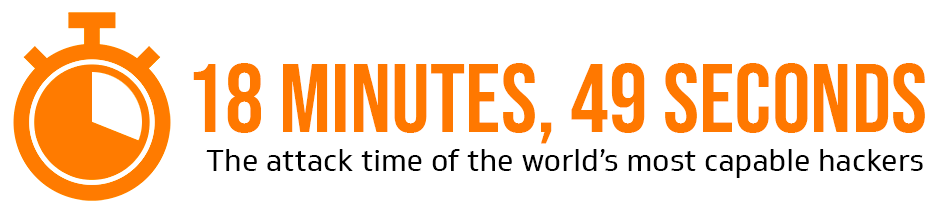
In the end, self-supervised AI platform users benefit from real-time threat detection that is, on average, three times faster than the attack time of the world’s most capable hackers (currently estimated at 18 minutes, 49 seconds), as compared to supervised platforms which typically detect novel attacks weeks or months later after human identification and investigation; or sadly, after the damage is done. With a Third Wave AI platform, concepts like “Negative Time to Detection” become possible.
The future of effective Cybersecurity defenses in a world where we must “Assume Breach” will depend on deployment of real-time detection capabilities built on Third Wave, Self-Supervised Artificial Intelligence.
Other MixMode Articles You Might Like
2022 Cybersecurity Statistics You Should Know About
New CISA Strategy Focused on Resilience, Inevitability of Data Breaches
Video: Modernizing Phoenix’s Cybersecurity to Combat Nation-State Attacks
Financial Services IT Teams Relying on Legacy Cybersecurity Plagued By False Positives and Negatives
Killware: How to Protect Your City From This Growing Next-Generation Cybersecurity Threat
Unsupervised Machine Learning: Benefits for the Financial Services Industry