Artificial Intelligence (AI) has been hailed as a game-changer in cybersecurity, promising to revolutionize threat detection, prevention, and response. However, as with any emerging technology, the hype surrounding AI has often outpaced its practical applications. Many security teams and CISOs have been burned by overblown claims and have become skeptical of AI-powered solutions.
The AI Oversell
In recent years, it seems like every cybersecurity vendor has been quick to slap the “AI” label on their offerings. But the reality is that many of these solutions are just advanced machine learning algorithms. While machine learning can be a powerful tool, it’s essential to differentiate it from true AI in a cybersecurity context.
Machine Learning vs. True AI
- Machine Learning: Machine learning algorithms can identify patterns in data and make predictions based on those patterns. They are trained on large datasets and can learn to recognize anomalies and potential threats.
- True AI: True AI, on the other hand, involves machines that can reason, understand, and learn independently. They can go beyond pattern recognition and exhibit human-like intelligence.
While both machine learning and artificial intelligence (AI) are powerful tools for cybersecurity, there are critical distinctions between them, particularly when it comes to threat detection.
Machine Learning: A Powerful Tool
- Pattern Recognition: Machine learning excels at identifying patterns in large datasets. It can learn from historical data to recognize anomalies and potential threats.
- Statistical Models: Machine learning algorithms make nuanced predictions and classifications using statistical models.
- Limited Understanding: While effective, machine learning lacks a deep understanding of the world as it has no contextual or reasoning capabilities, so can be limited in adapting to new or unexpected threats.
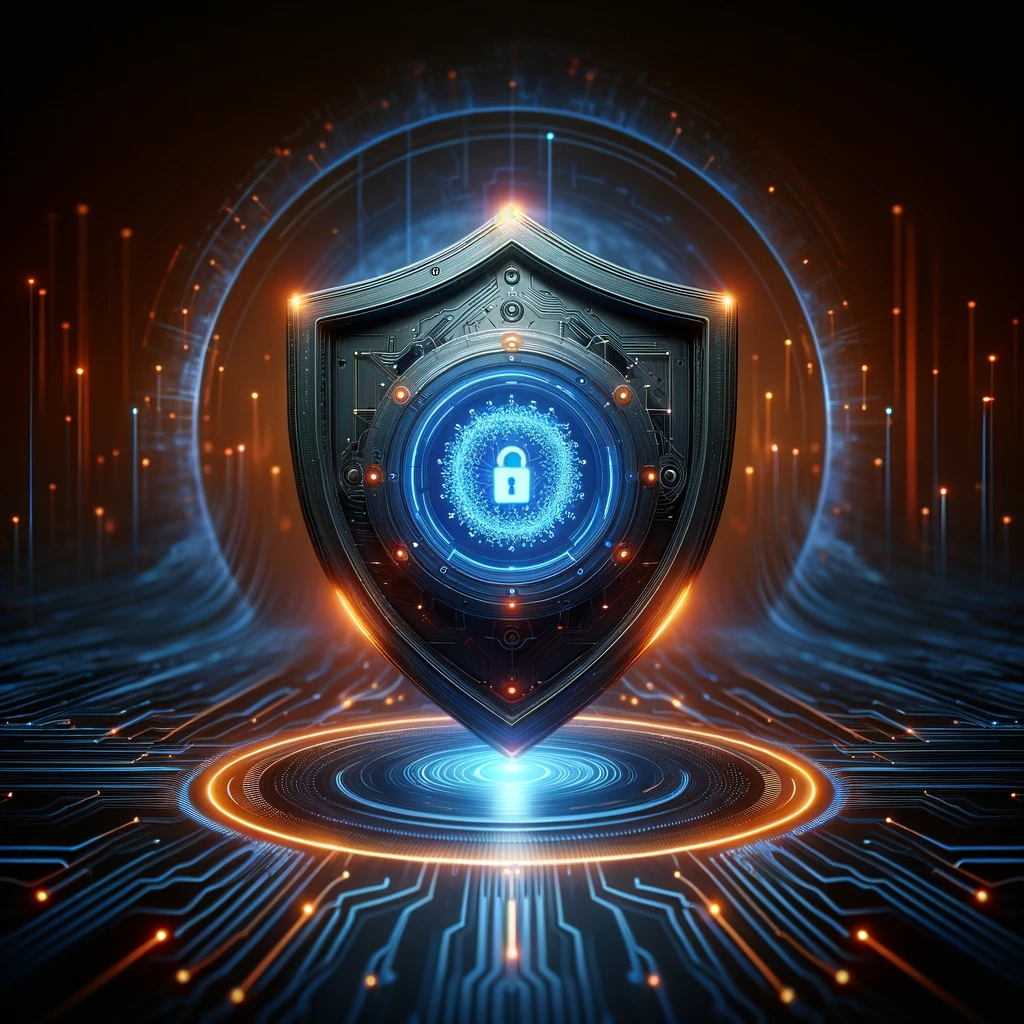
True AI: A Higher Level of Intelligence
- Reasoning and Understanding: True AI goes beyond pattern recognition. It can reason, understand, and learn independently.
- Adaptability: True AI solutions can adapt to new situations and learn from their experiences, making them more capable of handling unforeseen threats.
- Contextual Awareness: True AI can consider the context of a situation, making it more effective at detecting complex threats.
The Need for Critical Evaluation
Security teams and CISOs must approach AI-powered solutions with a critical eye. Refrain from being swayed by marketing hype or flashy demonstrations. Instead, focus on the following factors:
- Explainability: Can the AI system provide clear explanations for its decisions? This is crucial for understanding its limitations and building trust.
- Adaptability: Can the system learn and adapt to new threats as they emerge? A rigid system may struggle to keep up with the ever-evolving threat landscape.
- Data Quality: The data quality used to train the AI model is essential. Poor data can lead to inaccurate results and false positives.
- Human Oversight: AI should be used to augment human capabilities, not replace them. Human experts are still needed to provide context, make critical decisions, and address unexpected situations.
The Dark Side of the Black Box
Historically, many AI solutions have operated as black boxes, their decision-making processes shrouded in mystery. Security teams and CISOs have often relied on these opaque solutions without fully understanding how they arrived at their conclusions. This lack of transparency has led to several negative consequences:
- Loss of Trust: When AI solutions make unexpected or seemingly incorrect decisions, they can erode stakeholder trust. Without understanding the underlying logic, it becomes difficult to determine whether the system is functioning correctly or if there are underlying issues.
- Regulatory Compliance: Many industries, including finance and healthcare, are subject to strict regulations that require transparency and accountability. Black box AI systems can pose significant compliance risks if they cannot explain their decisions adequately.
- Limited Effectiveness: Without explainability, it is challenging to identify and address biases or errors in AI models. This can lead to suboptimal performance and missed opportunities to improve the system’s capabilities.
The Pros and Cons of the Black Box Approach
While black box AI systems have advantages, such as efficiency and scalability, they also have significant drawbacks.
Pros:
- Efficiency: Black box models can process large amounts of data quickly and efficiently, making them suitable for real-time threat detection.
- Scalability: These models can be easily scaled to accommodate growing datasets and increasing workloads.
Cons:
- Lack of Transparency: Black box models are inherently opaque, making it difficult to understand their decision-making processes.
- Limited Trust: This lack of transparency can lead to mistrust and skepticism among stakeholders.
- Regulatory Challenges: Black box solutions may not comply with regulatory requirements that demand transparency and accountability.
Why Explainability is Better
Explainable AI (XAI) addresses the limitations of black box models by providing insights into how AI solutions arrive at their decisions. By understanding the underlying logic, security teams can:
- Increase Trust: Explainable AI can foster trust among stakeholders by providing transparency and accountability.
- Improve Decision-Making: By understanding the factors influencing AI decisions, security teams can make more informed decisions and take appropriate actions.
- Enhance Efficiency: Explainability can help identify biases and errors in AI models, improving performance and reducing false positives.
- Meet Regulatory Requirements: Explainable AI can help organizations comply with regulations that require transparency and accountability.
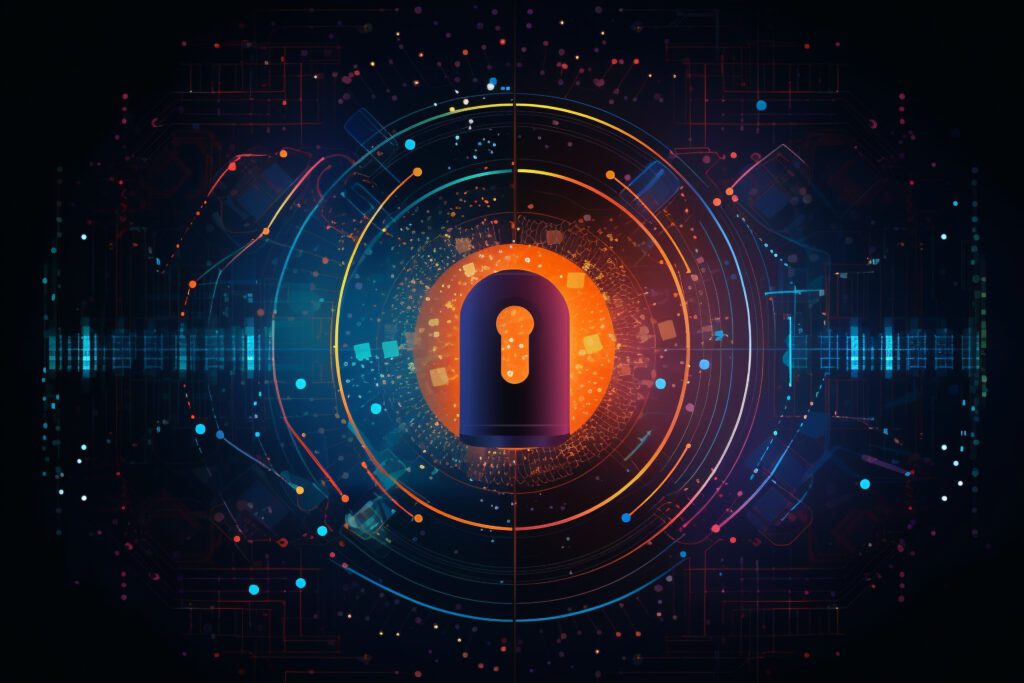
Critical Benefits of Explainability for AI-Driven Threat Detection
- Enhanced Threat Detection: Explainable AI can help security teams better understand the factors contributing to a threat, enabling them to develop more effective detection and response strategies.
- Improved Response: By understanding the root causes of incidents, security teams can take more targeted and effective actions to mitigate their impact.
- Reduced False Positives: Explainability can help identify and address biases in AI models, reducing the number of false positives and improving the overall accuracy of threat detection.
- Enhanced Decision-Making: Explainable AI can provide valuable insights to help security teams make more informed decisions about resource allocation, risk management, and incident response.
MixMode: The Leader in Third-Wave AI-Powered Cybersecurity
MixMode is leading the way in delivering true AI-powered cybersecurity solutions. As a pioneer in this field, MixMode’s AI is context-aware, adaptive and capable of reasoning, providing a unique combination of explainability, prioritization, and automation to help organizations stay ahead of emerging threats.
Explainability at the Core
One of MixMode’s key differentiators is its commitment to explainability. Unlike many AI-based cybersecurity solutions that operate as black boxes, MixMode provides clear and understandable explanations for AI decisions. This transparency is essential for building trust and ensuring security teams can leverage the Platform’s capabilities effectively.
How does MixMode achieve explainability?
- Contextual Understanding: MixMode’s AI models are designed to understand the context of security events, allowing them to provide more meaningful explanations.
- Visualization Tools: The MixMode Platform offers intuitive visualization tools that help users understand complex security data and the reasoning behind AI-driven decisions.
- Natural Language Explanations: MixMode can generate human-readable explanations of its findings, making it easier for security teams to interpret and act on the information.
Despite the challenges, artificial intelligence has the potential to make a significant impact on cybersecurity. As AI technology evolves, explainability is critical to AI-driven cybersecurity solutions.
Security teams can build trust, improve decision-making, and enhance their overall security posture by understanding how these AI-powered solutions arrive at their decisions. As AI plays a more significant role in cybersecurity, the demand for explainability will only grow.
Reach out to learn more about how our AI-powered solutions can help strengthen your security.
Other MixMode Articles You Might Like
Alarming Intrusion: Chinese Government Hackers Target US Internet Providers
Black Hat 2024 and the Rise of AI-Driven Cyber Defense
The Alert Avalanche: Why Prioritizing Security Alerts is a Matter of Survival
Gartner’s 2024 Hype Cycle for Zero Trust: Spotlight on Network Detection and Response
Critical Microsoft Zero-Day Vulnerability Exploited in the Wild for Over a Year