Gartner just released its Emerging Tech Impact Radar: Security, which looked at technologies that could help organizations effectively detect and respond to attacks and create better efficiencies through AI-based security hyper-automation.
One of the key areas the report calls out is Advanced Behavioral Detection Analytics, and highlights its importance for threat detection.
Advanced behavioral detection analytics refers to an emerging set of technologies that analyze the behavior and activities of users, systems, applications, and devices within a network or system. By establishing baseline patterns of normal behavior, deviations or anomalies can be identified, which may indicate potential security threats such as insider attacks, malware, or unauthorized access. Using machine learning and AI algorithms, these technologies can dynamically adapt and learn from new data, enhancing their accuracy in recognizing evolving threats that traditional rule-based methods would miss. Advanced behavioral detection analytics solutions not only aid in detection speed and confidence but also enable future prediction of threats based on proposed security control or network changes. Because adversaries will continue to advance their methods, the advancement of behavioral analytics engines must and will continue to progress.
Gartner, Inc. Emerging Tech Report 2023:Security
In today’s rapidly evolving threat landscape, organizations increasingly face the challenge of detecting and mitigating sophisticated cyberattacks. Traditional solutions, rule-based methods, and indicators of compromise (IOCs) are no longer sufficient to combat these threats. As a result, advanced behavioral detection analytics has emerged as a powerful and effective advanced threat detection solution.
Key Takeaways from the Gartner Report:
- Advanced Behavioral Detection Analytics: Advanced behavioral detection analytics refers to technologies that analyze the behavior and activities of users, systems, applications, and devices within a network or system. By establishing a baseline of user behavior, these technologies can identify deviations or anomalies that may indicate malicious behaviors and potential security attacks, such as insider threats, malware, or unauthorized access.
- Machine Learning and AI Algorithms: Advanced behavioral detection analytics leverage machine learning and AI algorithms to dynamically adapt and learn from new data. This enhances their accuracy in recognizing evolving threats and complex attacks that traditional security tools and rule-based methods would miss. These solutions not only aid in detection speed and confidence but also enable future prediction of threats based on proposed security control or network changes.
- Indicators of Behavior (IOBs): IOBs are based on the telemetry of the cyber behavior of users and network devices that can be tracked over time to detect anomalies. Threat-hunting solutions and processes utilizing IOCs and IOBs are the most effective in detecting novel attack models.
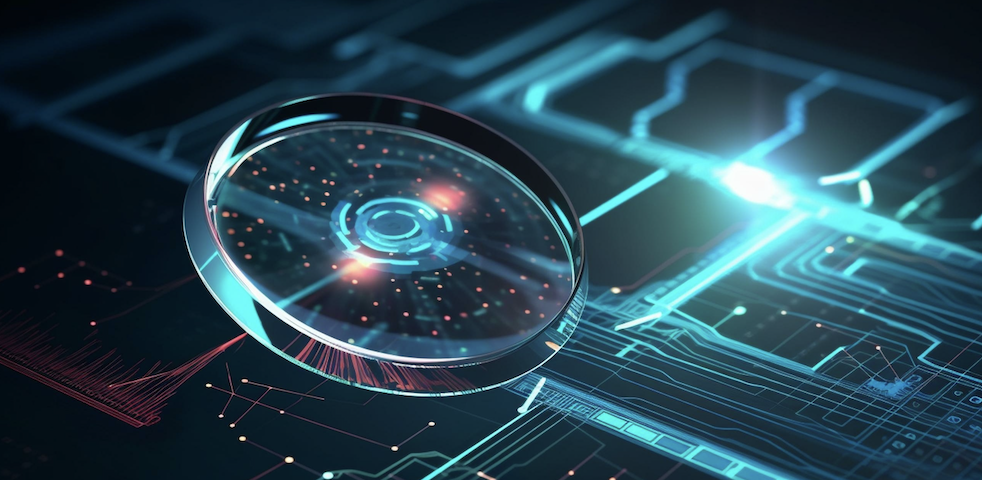
Importance of Advanced Behavioral Detection Analytics for Threat Detection:
- Enhanced Detection Accuracy: Advanced behavioral detection analytics provide a more comprehensive and accurate approach to threat detection. By analyzing behavioral indicators, security teams can identify subtle anomalies and potential threats that may go unnoticed by traditional methods.
- Proactive Threat Hunting: These advanced analytics enable proactive threat hunting by continuously monitoring and analyzing system and user behavior patterns. This proactive approach allows organizations to detect and respond to unknown attacks and advanced threats before they cause significant damage.
- Future Threat Prediction: By analyzing behavioral patterns and trends, advanced behavioral detection analytics can predict potential future threats. This empowers organizations to identify suspicious behaviors or abnormal activity to implement proactive security controls and network changes to mitigate cyber attacks before they materialize.
Legacy UEBA (User and Entity Behavior Analytics) platforms and AI-enhanced Advanced Behavioral Detection Analytics differ in several key aspects:
- Approach to Detection: Legacy UEBA platforms primarily rely on predefined rules and signatures to detect known threats and patterns. They analyze user and entity activities to identify deviations from normal behavior. In contrast, AI-enhanced Advanced Behavioral Detection Analytics leverages machine learning algorithms, anomaly detection, and behavioral analytics to identify patterns and anomalies that may indicate potential threats. They can detect evolving and unknown threats that may not have predefined rules or signatures.
- Data Analysis: Legacy UEBA platforms typically analyze structured data, such as logs and events, to detect anomalous activity. They focus on user activity, access patterns, and system events to perform behavioral analysis. On the other hand, AI-enhanced Advanced Behavioral Detection Analytics can analyze diverse data types and formats, including unstructured data such as text, images, and network traffic. They can apply machine learning algorithms to extract insights from this data and detect complex patterns and anomalies to uncover malicious activity.
- Automation and Intelligence: AI-enhanced Advanced Behavioral Detection Analytics platforms often incorporate automation and intelligence capabilities. They can automatically correlate and analyze large volumes of data, reducing the manual effort required for threat detection. These platforms can also leverage AI techniques, such as natural language processing and machine vision, to analyze unstructured data and extract meaningful information. Legacy tools and UEBA platforms may have limited automation capabilities and rely more on manual analysis and investigation to detect suspicious activity.
- Scalability and Performance: AI-enhanced Advanced Behavioral Detection Analytics platforms are designed to handle large-scale data processing and analysis. They can efficiently process and analyze massive amounts of data in real-time, enabling organizations to detect threats quickly. Legacy UEBA platforms may have limitations in terms of scalability and performance, especially when dealing with high volumes of data.
- Adaptability and Learning: AI-enhanced Advanced Behavioral Detection Analytics platforms can adapt and learn from new data and evolving threats. They can continuously update their models and algorithms based on the latest information, performing advanced analytics and improving their detection capabilities. Legacy UEBA platforms may require manual updates and configuration changes to adapt to new threats.
- Contextual Understanding: AI-enhanced Advanced Behavioral Detection Analytics platforms can provide a deeper contextual understanding of security events and incidents. They can analyze multiple data sources and correlate information to provide a holistic view of abnormal behavior and potential threats. Legacy UEBA platforms may have limitations in terms of contextual understanding, as they primarily focus on user and entity behavior without considering broader contextual factors.
Overall, AI-enhanced Advanced Behavioral Detection Analytics platforms offer more advanced and sophisticated capabilities than traditional solutions and legacy UEBA platforms when detecting real threats. They leverage AI and machine learning techniques to analyze diverse data sources, identify unusual behaviors, detect unknown threats, automate processes, and provide deeper insights for effective threat detection and response.
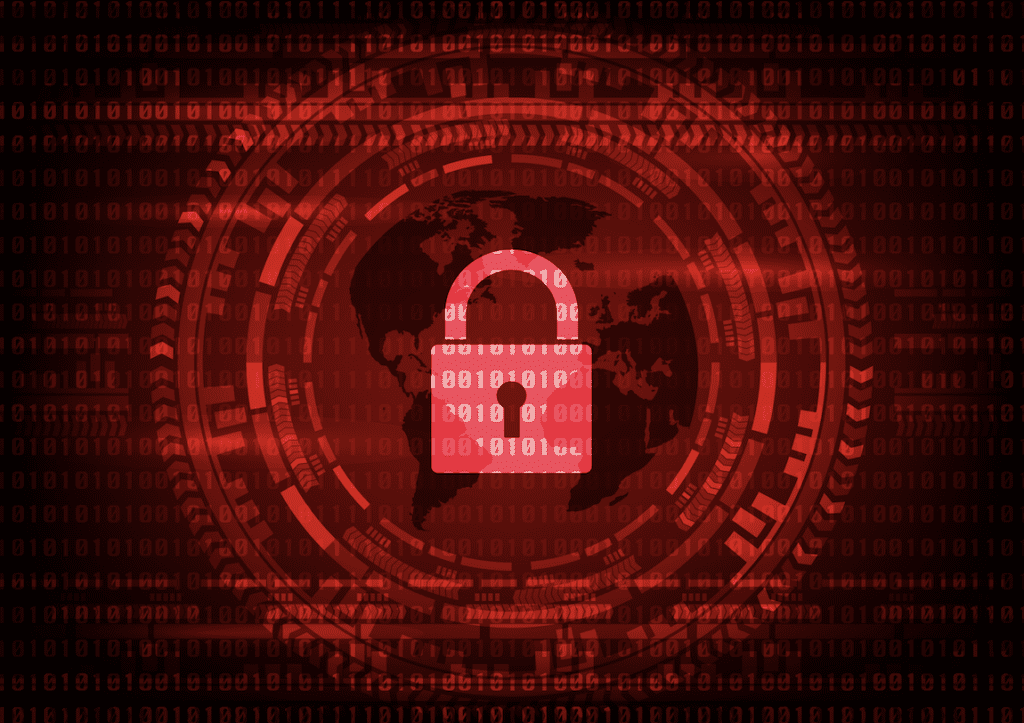
Using Advanced Behavioral Detection to Detect Ransomware
New malware variants are constantly emerging as attackers evolve their techniques, requiring defensive strategies to be continuously updated. Ransomware, due to the scale of disruption and costs, is one of the most serious and dangerous forms of malware that requires a stronger defense strategy. Advanced Behavioral Detection Analytics can play a crucial role in detecting ransomware by analyzing the behavior of various systems and users within a network. Here’s how ABDA helps in this context:
- Anomaly Detection: ABDA monitors the normal behavior patterns of systems, applications, and users within a network. It establishes a baseline of normal activities and identifies any deviations from this baseline. Ransomware often exhibits abnormal behavior, such as rapidly encrypting files or making unauthorized system settings changes. ABDA can detect these anomalies and raise alerts.
- File Activity Monitoring: Ransomware typically involves encrypting files on infected systems. ABDA can monitor file activity across the network, looking for suspicious patterns such as a sudden surge in file modifications or the creation of encrypted files. By analyzing these activities, ABDA can identify potential ransomware attacks.
- Network Traffic Analysis: Ransomware often relies on network communication to spread across systems or communicate with command-and-control servers. ABDA can analyze network traffic, looking for unusual communication patterns or connections to known malicious IP addresses. By detecting these indicators, ABDA can help identify ransomware-related activities.
- User Behavior Analysis: ABDA can also analyze user behavior to detect potential ransomware attacks. For example, if a user suddenly starts accessing many sensitive files or exhibits unusual login patterns, it could indicate a ransomware infection. ABDA can flag such behaviors and trigger further investigation.
- Machine Learning and AI: ABDA systems can leverage machine learning and artificial intelligence techniques to continuously improve their detection capabilities. By training on large datasets of known ransomware behaviors, ABDA can learn to recognize new variants or previously unseen attack patterns, enhancing its ability to detect ransomware in real-time.
Advanced Behavioral Detection provides a proactive approach to ransomware detection by monitoring and analyzing various behavioral aspects within a network. By identifying anomalies and suspicious activities, it helps organizations detect and respond to ransomware attacks more effectively.
Why MixMode
MixMode is a leading solution in the field of advanced behavioral detection analytics for several reasons:
- Real-time Threat Detection: MixMode utilizes advanced AI algorithms to analyze vast volumes of security telemetry data. This enables the detection of known and novel attacks in real-time, providing organizations with actionable insights to respond quickly and effectively.
- Self-Supervised Learning AI: MixMode’s Artificial Intelligence was born out of dynamical systems and utilizes self-supervised learning to achieve high detection accuracy. This helps cut through the noise and false-positive alerts to surface threats that matter.
- Continuous Learning and Adaptation: MixMode’s AI continuously learns from new data, adapting its detection capabilities to each network and the evolving threat landscape. This enables organizations to stay ahead of emerging attack vectors and identify possible threats before they become full-blown attacks.
- Guided Response: MixMode surfaces high-fidelity alerts with guided response recommendations integrated with threat intelligence and The Mitre ATT&CK Framework. This ensures that organizations can quickly and effectively respond to new threats.
MixMode is leading the charge for real-time threat detection of known and novel attacks at scale. By leveraging advanced behavioral detection analytics, security teams can get the behavioral detection capabilities and detailed insight needed to strengthen their security posture and effectively combat the ever-evolving threat landscape.
Reach out to learn more or download the Gartner Emerging Tech Impact Radar: Security report.
Other MixMode Articles You Might Like
The Importance of Real-Time Threat Detection at Scale: Unveiling the Hidden Attack Surface
Insights and Trends from Gartner Emerging Tech Impact Radar: Security
Buyers Guide for AI Threat Detection and Response
Utilizing Artificial Intelligence Effectively in Cybersecurity
Bridging the Gaps: Why ITDR is the Missing Link in Identity Protection