
Gartner recently released its report Emerging Tech: Top Use Cases for AI in Threat Detection, Investigation, and Response (TDIR), highlighting how security leaders utilize artificial intelligence (AI) to enhance detection, streamline investigations, and automate response to cyber threats. With increasingly frequent and sophisticated attacks, AI represents a crucial opportunity to augment human security teams.
Key Findings
Among the key findings are:
- More than 50% of interviewed security providers already use supervised machine learning (ML)-based AI to enhance their threat detection capabilities.
- The use of AI by malicious parties is accelerating the evolution of their attack capabilities and compelling security product leaders to adopt and implement AI technologies more quickly to respond with improved threat detection, investigation and response (TDIR) solutions.
What is TDIR?
TDIR refers to the cybersecurity processes for threat detection, investigation, and response. This includes identifying threats through monitoring, analyzing suspicious activity to determine if it’s malicious, investigating threats to understand scope and impact, and executing responses to contain and remediate confirmed incidents.
Top AI Use Cases for TDIR
The report outlines the top use cases for TDIR, including:
Detection
Over 50% of providers are leveraging supervised machine learning to identify threats and patterns at scale beyond human capacity. AI can pinpoint emerging attacks early by combining signals across endpoints, networks, and other security tools. However, some models still require expert guidance for training and output validation.
Triage
Security teams often spend more time investigating false positives than responding to incidents. AI can help automate the triage process to filter out irrelevant alerts so analysts can focus where needed.
Investigate
AI assistants can compile relevant data and context to create investigation-ready cases in minutes versus hours. Analysts are freed from manual busywork to concentrate on higher-level assessments and questions for which AI chatbots can parse and provide recommendations.
Respond
Predefined response playbooks will allow AI engines to execute containment workflows autonomously to isolate and mitigate confirmed threats with speed and consistency. However, security practitioners’ reviews remain essential for approving actions for effective threat response.
What is Unsupervised Machine Learning
The report showed that more than 50% of interviewed security providers claim to already use supervised machine learning (ML)-based AI to enhance their threat detection capabilities. While an improvement, it still has limitations.
Unsupervised machine learning is a type of machine learning where the model learns from unlabeled data, seeking to identify patterns, structures, or anomalies within the data without explicit guidance or supervision. In unsupervised learning, the algorithm explores the data to find hidden insights or intrinsic structures, such as clusters or associations, without being explicitly told what to look for.
On the other hand, artificial intelligence (AI) is a broader concept that encompasses the simulation of human intelligence processes by machines, including learning, reasoning, problem-solving, perception, and language understanding. AI includes various subfields, such as machine learning, natural language processing, computer vision, and robotics.
Therefore, unsupervised machine learning is a subset of AI, specifically focusing on learning from unlabeled data to uncover patterns or structures. At the same time, AI encompasses a broader range of technologies and techniques to simulate human intelligence in machines.
Why is Unsupervised Machine Learning Not Good for Advanced Detection?
Unsupervised machine learning, while valuable for detecting anomalies and patterns in data, is not always sufficient for advanced threat detection due to several reasons:
- Lack of Context: Unsupervised machine learning models may struggle to contextualize the anomalies they detect within the broader environment. Without understanding the context in which anomalies occur, it can be challenging to distinguish between benign anomalies and genuine security threats.
- Interpretability: Unsupervised machine learning models often lack interpretability, making it difficult for security analysts to understand why a particular anomaly was flagged as a potential threat. This can hinder the ability to effectively investigate and respond to security incidents.
- Evolving Threat Landscape: Advanced threats are constantly evolving, and unsupervised machine learning models may struggle to adapt to new and emerging threat patterns without human intervention or labeled training data.
- False Positives: Unsupervised machine learning models may generate many false positives, leading to alert fatigue and potentially diverting attention away from genuine security threats.
- Adversarial Attacks: Unsupervised machine learning models can be vulnerable to adversarial attacks, where threat actors deliberately manipulate data to evade detection by the model.
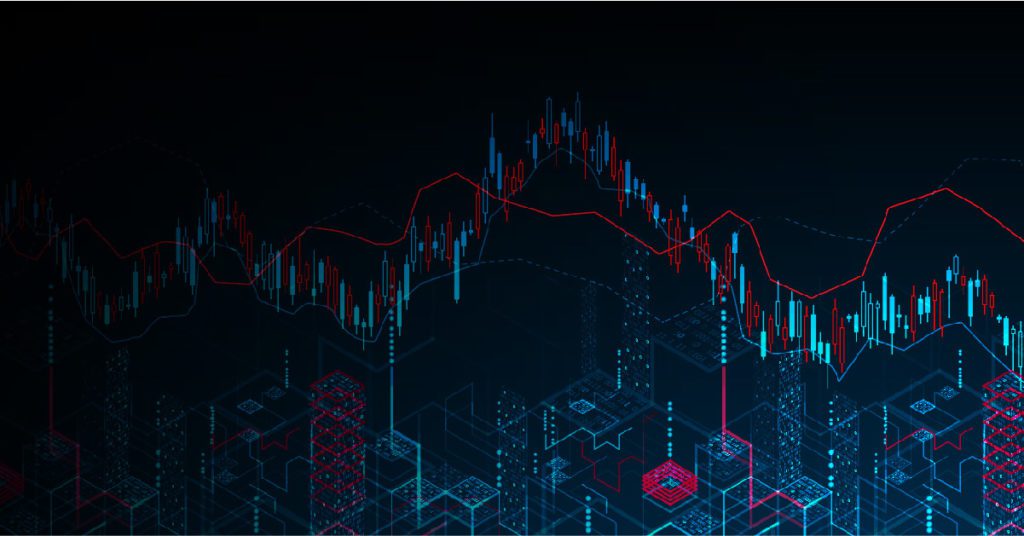
Utilizing Self-Supervised Learning for Advanced Detection
Self-supervised learning is a type of machine learning where the model learns from the data itself without requiring explicit labeling of the data by humans. In self-supervised learning, the algorithm generates its own labels or supervisory signals from the input data, often by creating a pretext task the model tries to solve. This approach allows the model to learn from vast amounts of unlabeled data, making it more scalable and adaptable to diverse datasets.
Self-supervised learning is particularly well-suited for advanced threat detection for several reasons:
- Adaptability: Self-supervised learning models can adapt to new and evolving threat patterns without needing large amounts of labeled training data. This adaptability is crucial in cybersecurity, where threats are constantly changing.
- Anomaly Detection: Self-supervised learning can be used to identify anomalies or deviations from normal patterns within the data, which is essential for detecting advanced and previously unseen threats.
- Contextual Understanding: By learning from the data, self-supervised models can develop a deeper contextual understanding of the environment in which they operate, enabling them to distinguish between benign and malicious activities more effectively.
- Reduced Dependency on Labeled Data: Traditional supervised learning approaches often require large volumes of labeled data, which can be challenging to obtain in cybersecurity. Self-supervised learning reduces this dependency, making it more practical for threat detection in real-world scenarios.
The AI Security Imperative
Attackers are also tapping AI to boost their capabilities. Organizations must fight back with AI-enabled defenses to keep pace with constantly evolving threats. AI can ingest signals across a company’s digital footprint to detect novel attack patterns and vulnerabilities missed by traditional tools.
How AI Helps
Gartner outlined several reasons how AI could help, including:
- Analyze signals across digital ecosystems at scale impossible for humans
- Accelerate processes to respond to threats faster
- Reduce menial tasks for analysts to focus their expertise on critical thinking
- Continually detect new threat patterns and vulnerabilities missed by traditional tools
Cautious Adoption
Despite rapid advancement, providers are rightly cautious about potential data exposure, output accuracy, and unintended impacts from AI assistance. Strict data governance, internal vetting, and human validation policies are imperative.
Realizing the Potential
For security leaders, the report emphasizes that AI integration must focus on making experts more effective, not replacing them. Targeted AI that removes tedious tasks allows for more impactful expert contributions. Failing to utilize AI may soon mean failure to defend against AI-powered threats.
Product leaders seeking to implement AI in the threat detection process need to understand that the end goal is not about replacing human involvement; it is about making security experts more effective. It is also to be focused on improving the retention of analysts by making the work they do more engaging and less mundane. – Gartner
Gartner’s Recommendations
Gartner recommends organizations:
- Include AI models in your threat detection solutions that can learn from large datasets and are capable of being trained by experienced security experts who know how to detect advanced attack methods.
- Develop a plan that details how and where you intend to use generative AI (GenAI) to assist your clients with threat triage, investigation, and response capabilities.
- Determine which areas of the TDIR process require the most immediate integration of AI to counter and combat threat actor adoption of AI.
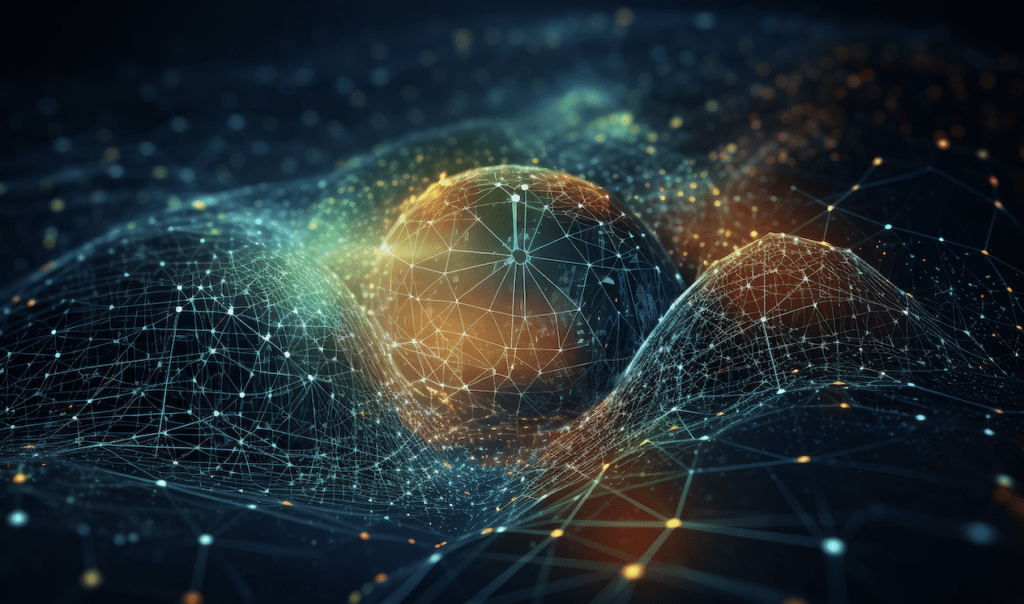
Building a Future-Proof Security Posture with MixMode AI
In the digital age, where attack surfaces are vast, and threats evolve constantly, organizations need a proactive and intelligent approach to security. Traditional methods struggle to keep pace, leaving them vulnerable and exposed. This is where MixMode steps in, not as a replacement but as a powerful force multiplier, leveraging the potential of AI to revolutionize threat detection, investigation, and response.
MixMode’s advanced AI capabilities offer demonstrable advantages:
- Unparalleled Threat Detection: MixMode’s self-supervised learning algorithms analyze vast volumes of security telemetry, identifying subtle anomalies and suspicious activities that traditional methods miss. This early warning system allows for swift intervention of actual threats before they can escalate.
- Streamlined Investigations: The MixMode Platform automates the initial phases of incident investigation, correlating data, prioritizing alerts, and extracting actionable insights. This empowers security teams to focus on complex analysis and decision-making, significantly reducing investigation time and effort.
- Guided Response: The MixMode Platforms built in threat intelligence and Mitre ATT&CK Framework empowers security analysts with the comprehensive threat insights needed. This ensures a swift and decisive response, even when resources are limited.
MixMode offers a strategic advantage:
- Continuous Learning and Adaptation: MixMode’s AI continuously learns from new data and emerging threats, adapting its detection algorithms to stay ahead of the curve. This proactive approach ensures long-term effectiveness and minimizes the risk of future vulnerabilities.
- Seamless Integration: The MixMode Platform integrates seamlessly with existing security infrastructure, leveraging data sources and workflows. This avoids costly rip-and-replace scenarios and ensures a smooth transition to AI-powered security.
- Empowered Security Teams: The MixMode Platform empowers security teams by providing them with actionable insights, threat intelligence, and automated capabilities. This frees them from tedious tasks and allows them to focus on strategic analysis and proactive threat hunting.
MixMode leverages the power of AI to build a future-proof security posture, enabling organizations to detect threats earlier, investigate them faster, and respond more effectively. By embracing MixMode, organizations can confidently navigate the ever-changing threat landscape and build a resilient security foundation for the digital age.
Download the report to learn more about Gartner’s insights into using AI for TDIR, or contact us today to discover how AI can revolutionize your security operations.
Other MixMode Articles You Might Like
Fighting Fire with Fire: AI and the Battle Against Ransomware
Defense-in-Depth: A Comprehensive Approach to Modern Cybersecurity
Overcoming Cloud Security Challenges: The Power of Cloud-Native AI-Driven Solutions