In Louis Columbus’s article, “Machines Protecting Themselves Is The Future Of Cybersecurity,” he proposes that modern cybersecurity breach attempts are succeeding in misdirecting human responses, which creates a great need for machines to be able to protect themselves.
His point has been particularly evident throughout the COVID-19 lockdown due to an influx of breaches, phishing attempts, malicious websites and hacks. Natural disasters, acts of terror, and global disasters are always followed by a massive rise in all methods of cyber attack, and the world needs to be prepared.
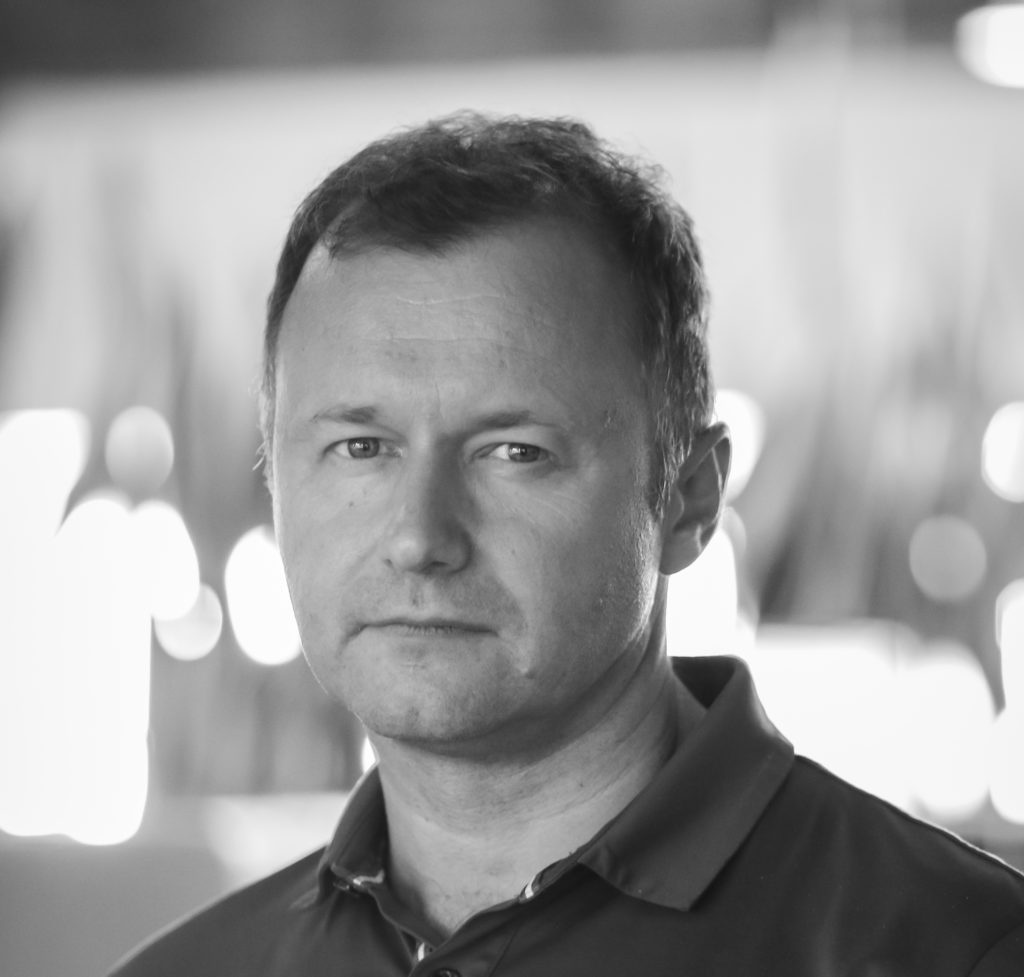
Columbus’s article highlights that during his keynote at the 2017 Institute for Critical Infrastructure Technology, General Hayden of the Department of Defense made a call to action to the private sector to take the initiative and innovate quickly to strengthen our cybersecurity.
We interviewed MixMode CTO Dr. Igor Mezic to better understand how machines should be protecting themselves with AI, what roles humans play, and how the DoD and the private sector both benefit from rapid innovation in cybersecurity.
Why do you think General Hayden believes that machines are more capable of protecting themselves than humans are at protecting them? What weaknesses do humans have that machines do not?
I think the most important part is that the amount of data that goes over a network on a typical machine is just beyond human capability to address. Treats could be hiding pretty much anywhere in that data so ultimately it’s not even an opinion, it’s just a fact that humans are less capable of processing that amount of data. On the other hand, humans are very intuitive so they are able to understand the changes in the data better than the current versions of machine intelligence can. So it’s a tradeoff. We need to develop the human-like intuitive ability to understand changes on a network from massive amounts of data. That combination is what is really interesting and important.
Why is it important for a machine to be able to protect itself?
Well, there are all kinds of threats that are showing up in the outside world, so at the end of the day, the machine has a purpose. It needs to satisfy certain needs that humans in an organization are expecting from it, but it cannot fulfill that purpose if it is being constantly attacked and prevented from executing.
Imagine an organization that has over 1000 computers. How many humans would you need to observe all of them for 24 hours every single day to catch if something was going wrong with them or why they weren’t working at an optimal pace? This is not just security. It’s beyond that. It’s trying to understand whether the machines are actually working optimally just like a manager would want to ensure that their human employees were working at an optimal pace as well.
How does AI fit into this equation? Can all types of AI aid in this?
AI fits in to start with because it’s an overlay on an immense data processing capability. It’s a learning layer.
We started with rule-based AI configurations and those are not extremely powerful in giving us what we want because they unfortunately ended up having a massive amount of false positives and negatives. So, it’s this issue of having an enormous amount of data but really separating the good from the bad. Then we started using learning algorithms, but they still needed a huge amount of labels to help them determine what is good and what is bad. Ultimately, now we are going towards algorithms that can recognize on their own what is going on.
If you think about the interaction between machines and humans in an optimal sense the labeling itself requires an enormous amount of human time. You need to collect the labels, put them in the files, and feed them to the learning system. With unsupervised or self-learning, to start with, you do a lot of unsupervised ingestion and learning. Then there is a little supervision as you go on, a small amount of effort from the human, which can act like a parent explaining how to categorize things. But it minimizes the effort needed from a human to teach the AI system and therefore optimizes the interaction.
Is one type of cybersecurity better than another in detecting breaches?
What kind of cybersecurity system should companies be employing to best defend from cyberattackers?
Unsupervised Learning can be both discriminative and generative. For example, unsupervised can mean clustering, which is not the same thing as generative or self-learning. Generative really references the fact that it can learn and then kind of predict what is going to happen in the next period of time, or what the next data point is going to look like, from the data you have.
Generative Unsupervised Learning is the most capable type of AI system available in terms of detecting zero-day attacks because it does not rely on previously labeled data to be able to recognize that something is going wrong on the network. Generative Learning AI, like what we have at MixMode, can predict what the network should look like at any time so it is able to -catch an anomaly when it appears.
General Hayden made a call to action to the private sector to innovate a solution to our cybersecurity woes. How can the private sector aid in strengthening cybersecurity in the US?
Developing an AI infrastructure that makes systems more safe of course benefits the private sector, but it also benefits the Department of Defence (DoD). The DoD, arguably, is under a lot of stress on the cybersecurity side. It demands systems that are at the higher potency level than the standard systems that are being used. So while smaller private orgs might require medium tier solutions – something that keeps them safe but is not super intelligent – orgs like the DoD really need very high end AI.
It’s like Volkswagen versus Ferrari. In many areas of the private sector you can get away with a Volkswagen, but you’re going to want a Ferrari in the DoD. It’s very similar in the case of large banks or medical systems. Nobody in a large medical system wants their patients’ data stolen. The way we can help is by developing the most cutting edge technologies that are really quite ahead of what the adversaries can do. There is a typical defense posture that the DoD actually uses which says, “I need to be some years ahead of my adversary so I have a guaranteed win.” In other words, “So that I don’t get a breach to such a large extent that national secrets are in limbo.” Clearly for that we need superior AI that our adversaries don’t have but that at the same time serves the private sector like large banks.
What is MixMode doing specifically to aid in this call to action?
Our goal is to introduce Third Wave Generative Unsupervised AI models into the market in a powerful way. We are already talking to the DoD and a variety of places to deploy it, but we are on our way to be able to help with those very high level AI needs and I think that puts us pretty far ahead in this space.
How are AI powered cybersecurity tools making detecting breaches easier?
To start with, if you look at our own strategy in addressing this, it is to reduce the amount of time that an analyst spends on detecting a breach. The way we’ve done that is by creating AI that is able to point out an interval in time in which something unusual has happened and bring to the analyst’s view maybe the top three machines that were involved in the odd activity.
If someone wants to go further, in our platform they have all the detection, intels, and notices that showed up all the way down to the raw packets so that they can go really deep. We bring them to this point in time and to the machines that might have been involved. We are currently building this root-cause analysis that will also give organizations a hypothesis of what really happened. That’s something we are in the middle of developing. At this point in time we really reduce a massive amount of their time, like 90%, that was previously spent looking at things that are not worth looking at because the AI narrows their attention to a focused set of intervals that are worth looking into.
MixMode Articles You Might Like:
Supporting Cybersecurity Programs throughout the Covid-19 Crisis
New Video: Does MixMode work in the cloud, on premise, or in hybrid environments?
IDC Report: MixMode – An Unsupervised AI-Driven Network Traffic Analysis Platform
MixMode Raises $4 Million in Series A Round Led by Entrada Ventures
In Case You Missed It: MixMode Whitepapers & Case Studies
In Case You Missed It: MixMode Integrations of 2020
New Video: How does MixMode’s context-aware AI build a network baseline?