Mixmode’s platform utilizes unsupervised AI (also known as context-aware or third-wave AI) to inform the user of threats (including Zero-Day, and encrypted traffic) on their network, and reduces the rate of false positives in intel and alerts consistently by 90% or more. In addition to being efficient, one of the key characteristics of the design is that it is transparent.
Here we describe the type of information that Mixmode’s AI system “looks” at for decision-making, and emphasize how it is human-like in behavior but with a substantially enhanced computational capacity.
At the core of the system are network modes – patterns that represent common behavior on the network over a specific timescale. In Figure 1 we show such a pattern. On the horizontal axis are integer labels for source IP’s, while on the vertical axis we show integer labels for destination IP’s.
The timescale represented in Figure 1 is that of 24 hours, and thus the colors in it represent daily interaction.
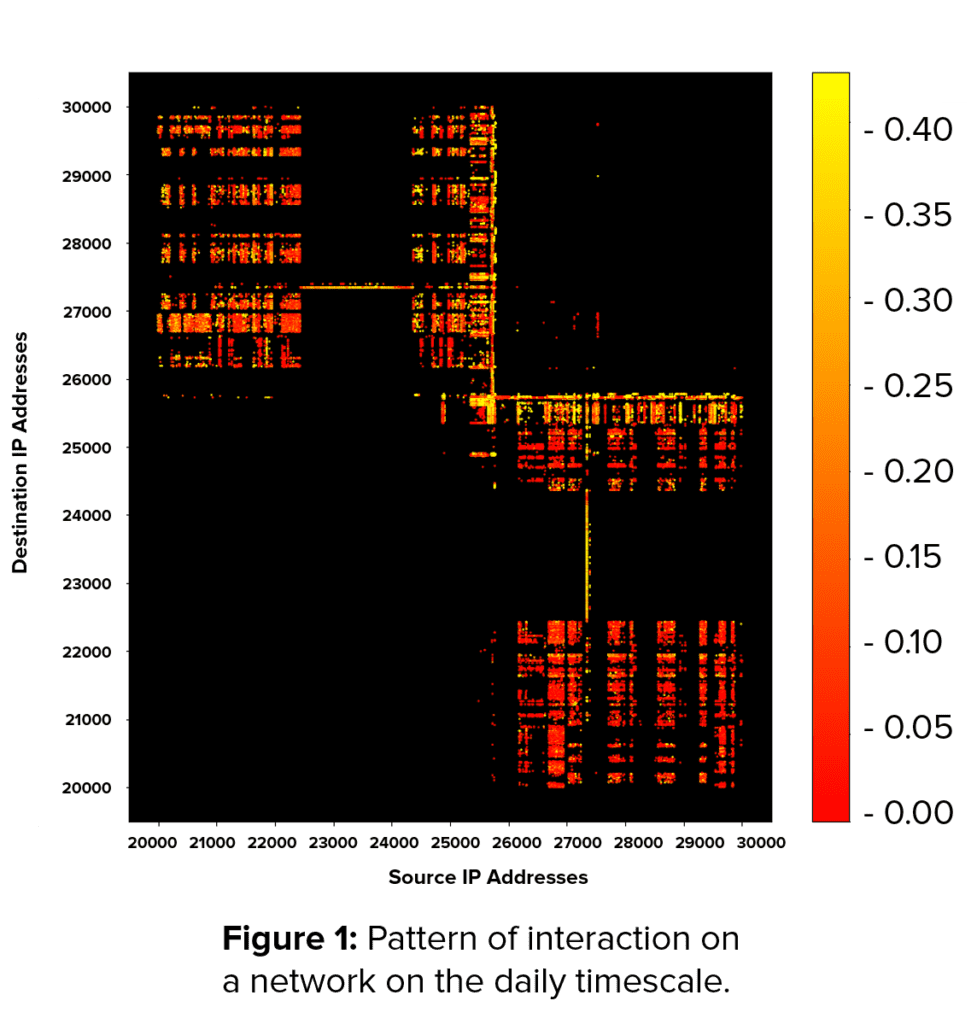
It is useful to look at the intersection of lines emanating from a specific source IP and destination IP.
Colors on the vertical line stemming from a specific label, say 21000, on the horizontal axis indicate the level of data flow from that IP to any destination IP represented on the vertical axis.
Colors on the horizontal line stemming from a specific label, say 22000, on the vertical axis indicate the level of daily flow to that IP from any source IP represented on the horizontal axis.
For example, the source IP labeled 21000 and the destination IP labeled 22000 do not interact on a daily timescale as evidenced by the black color at the intersection of the vertical and horizontal lines stemming from them. However, if the color is yellow, the level of activity is high.
The information that Mixmode’s AI analyzes consists of many such modes representing activity on many different timescales. But, even the single “heatmap” shown in Figure 1 contains a lot of information about the network:
- It is clear that source IP’s labeled roughly 21000-25000 only send information to destination IP’s labeled roughly 25700-26000, and vice versa. This immediately indicates two different subnetworks of this network that can be easily identified by Mixmode’s AI.
- There is also some deviation from that pattern. A few scattered dots in the upper right and lower left corner indicate there is some interaction within individual subnetwork.
Mixmode’s AI computes such patterns of interaction over many different timescales, and contrasts the pattern over the next short interval of 5 minutes with what was seen previously. If the patterns deviate, an assessment of the security risk implied in the deviation is computed and presented to the user. In addition, if the risk is low, the deluge of intel and notices presented to the user is minimized, eliminating false positives. Finally, the baseline of the network is built and adjusted automatically on an ongoing basis. This eliminates the need for ongoing tuning as with most other security platforms.
Thus, Mixmode’s third-wave AI makes its decisions on Zero-Day threats and False Positives based on the intuitively transparent concept of interaction of network elements over a variety of timescales – in the same way a human would – but utilizes its massive computational powers to do it efficiently.
Dr. Igor Mezic is the Chief Scientist and CTO for MixMode. He has spent his career developing highly complex algorithms and artificial intelligence for data analytics . He graduated with a doctorate from CalTech, holds 5 patents, and is a professor of mechanical engineering at the University of California, Santa Barbara. The MixMode AI, featuring algorithms used in projects with DARPA and the DoD is the first commercial use of true third-wave AI.
MixMode Articles You Might Like:
Turning the Unsupervised Tables on the Turing Test
Featured MixMode Client Success Story: HighCastle Cybersecurity
Top 5 Ways AI is Making Cybersecurity Technology Better
What is Network Detection and Response (NDR)? A Beginner’s Guide
The Tech Stack Needed to Start an MSSP Practice: Firewall, SIEM, EDR and NDR
AI-Enabled Cybersecurity Is Necessary for Defense: Capgemini Report