Across the Cybersecurity marketplace, vendors promise solutions to meet the modern needs of a large enterprise’s sprawling hybrid network. Phrases like “end-to-end coverage” and “real-time alerting” may sound promising, but too many vendors leave out critical information about the inherent limitations of their products.
In truth, many of these tools — especially those sold as part of bundled packages — fall very short when it comes to comprehensive cybersecurity and real-time alerting at an adequate scale, leaving open critical security gaps and limiting network traffic visibility.
The main challenge many of the cybersecurity products on the market today struggle to overcome is the difficulty of monitoring multi-cloud environments. Many are only capable of handling pieces of one environment rather than giving an enterprise visibility across all of their environments. This leaves costly gaps in their security posture and significantly increases the likelihood of an attack.
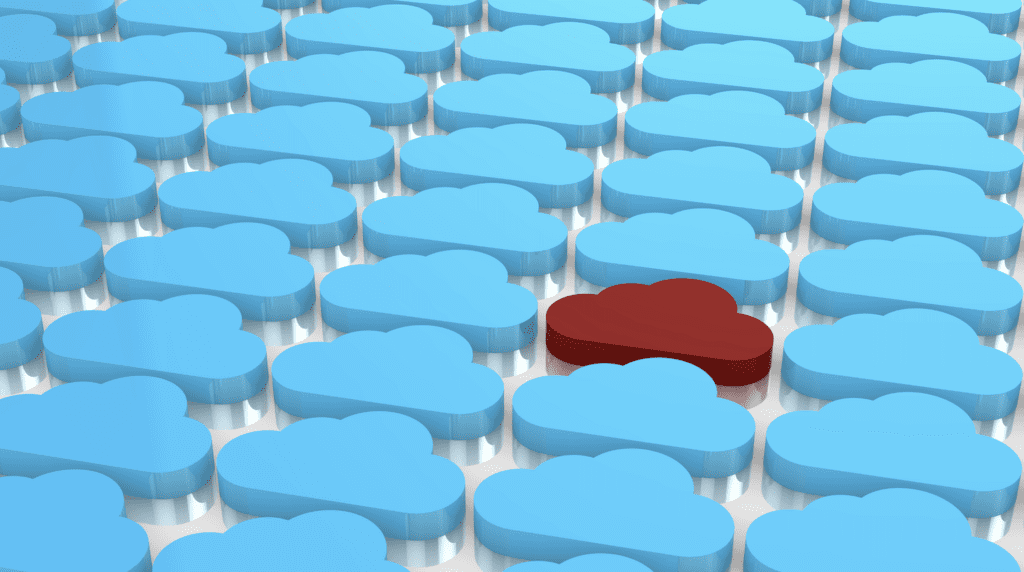
Multi-Cloud Environments Introduce Visibility and Coverage Challenges
Large, scalable networks today tend to rely on multi-cloud environments (including public cloud environments) to varying degrees, but getting a handle on visibility and performing real-time threat alerting across multi-cloud environments present several challenges.
- First, the bundled security tools that come with public cloud licenses are simply not built for real-time alerting at scale. As enterprise networks grow, the basic tools available through public cloud resources can’t keep up. Gaps and lags emerge as network traffic expands — it’s not a problem growing, successful enterprises want to encounter. A security solution capable of growing alongside a healthy company is money in the bank.
- Second, while individual cloud service providers typically offer oversight tools, comparing and contrasting cloud traffic across disparate systems and measurements is exceedingly difficult. When visibility is segmented, it’s nearly impossible to develop a comprehensive, centralized strategy given the complexity of trying to normalize data into a common format. Instead, SOCs frequently switch between cloud service provider interfaces and often feel less than confident about the level of full network traffic visibility.
As shared in our series on improving the black box approach to cybersecurity, customers are forced to consider business problems based on the data available to them. The reality is that data stored in multiple data formats — cloud, log, or network data — is inherently challenging to analyze. Customers want to solve problems like identifying and understanding anomalies or account access behaviors by correlating anomalous behaviors of specific accounts with other parameters like geography or ingress and egress points, but few rules-based Cybersecurity tools have the ability to do that without a great deal of manual data massaging and manipulating.
Customers have been forced to extract, transform and normalize data from multiple data sources and add to it or manually correlate it in order to pull out relevant information about anomalous behavior.
This set of circumstances is a common and significant industry-wide problem.
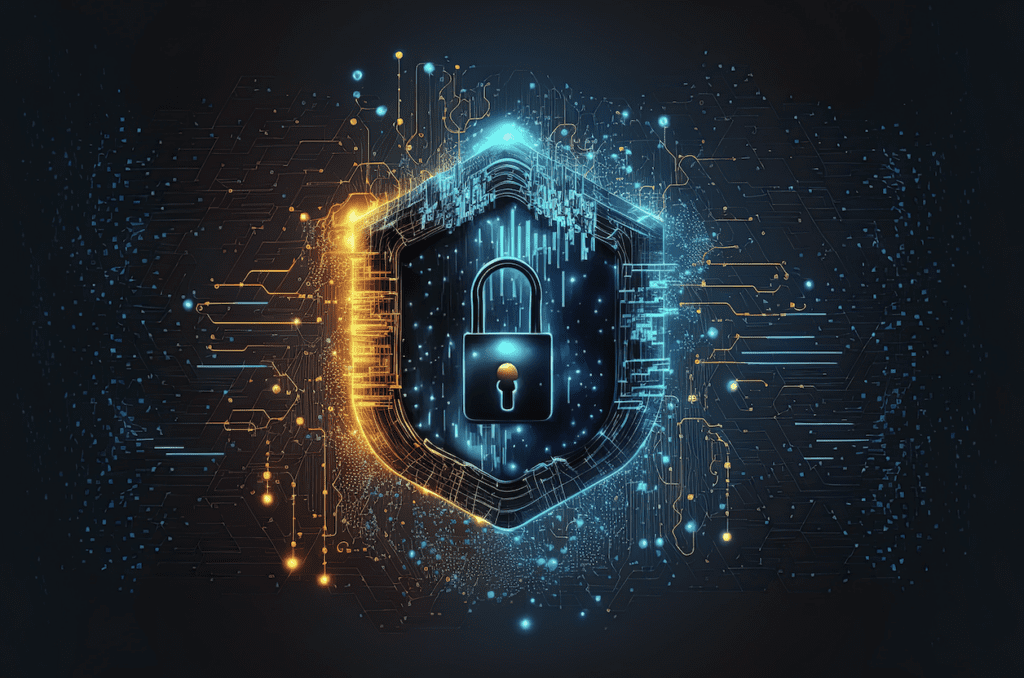
Evolution in Securing Large, Complex Data Environments
MixMode uncovers the gaps outlined above in almost every meeting we have with large enterprises.
Let us be clear: these gaps don’t exist because large enterprises are ignoring these specific environments, they exist because legacy platforms fail to handle the scale of these platforms with precise, real-time alerting at a reasonable cost. Furthermore, the bundled tools they are depending on are not optimized for security, have built-in gaps and only handle pieces of one environment rather than giving an enterprise visibility across all of their environments.
MixMode delivers a patented, self-learning platform that acts as the Cybersecurity Intelligence LayerSM to detect both known and unknown attacks, including novel attacks designed to bypass legacy cyber defenses dependent on rules and threshold-based detection.
This is accomplished in real-time, across any cloud or on-premises data stream (any machine-generated data: firewall, EDR, logs, syslog, API events, flowlog, Cloudtrail, raw network traffic layer 1-7, OT, etc).
No data formatting, ETL, or proprietary storage is required.
MixMode’s proprietary Third-Wave AI relies on a self-supervised learning methodology capable of understanding that the environment is changing based on the contextual information it observes. It observes the totality of the information available to it from the network sensor and detects any unusual behavior, be it on the lateral movement, inbound traffic or outbound traffic.
Learn more about how MixMode’s Third-Wave AI outperforms legacy and bundled cybersecurity tools, offering comprehensive visibility and real-time alerting across large-scale enterprise networks.
Other MixMode Articles You Might Like
What is the National Cybersecurity Strategy?
SANS First Look Report: Self-Supervised Learning Cybersecurity Platform for Threat Detection
Top Data Breaches in 2022 and 2023 Point to Increases in Phishing and Ransomware
Webinar On-Demand: Meeting Federal Zero Trust Requirements with Third Wave Artificial Intelligence
8 of the Biggest Ransomware Attacks in Recent History: A Look Back