The terms “machine learning” and “artificial intelligence” are frequently used in cybersecurity, often interchangeably, leading to confusion about their precise meanings and applications. Both machine learning and artificial intelligence play pivotal roles in fortifying cybersecurity defenses, yet they encompass distinct methodologies and applications. What are the disparities between them? And how do these technologies converge to bolster cyber resilience?
Understanding the Essence: Machine Learning vs. Artificial Intelligence
Machine Learning: Unveiling the Power of Data-Driven Learning
Machine learning is a subset of artificial intelligence that enables systems to learn and improve from experience without being explicitly programmed. At its core, machine learning revolves around using algorithms and statistical models to allow computers to perform tasks and make predictions based on patterns and inferences derived from data. This iterative learning process empowers machines to discern patterns, recognize anomalies, and make data-driven decisions, enhancing their efficacy over time.
Artificial Intelligence: The Pursuit of Human-Like Intelligence
Artificial intelligence, on the other hand, encompasses a broader spectrum of technologies and methodologies to enhance machines with human-like cognitive abilities, such as reasoning, problem-solving, and decision-making. While machine learning constitutes a fundamental component of artificial intelligence, the latter encompasses additional disciplines, including natural language processing, knowledge representation, and symbolic reasoning, with the overarching goal of simulating human intelligence within machines.
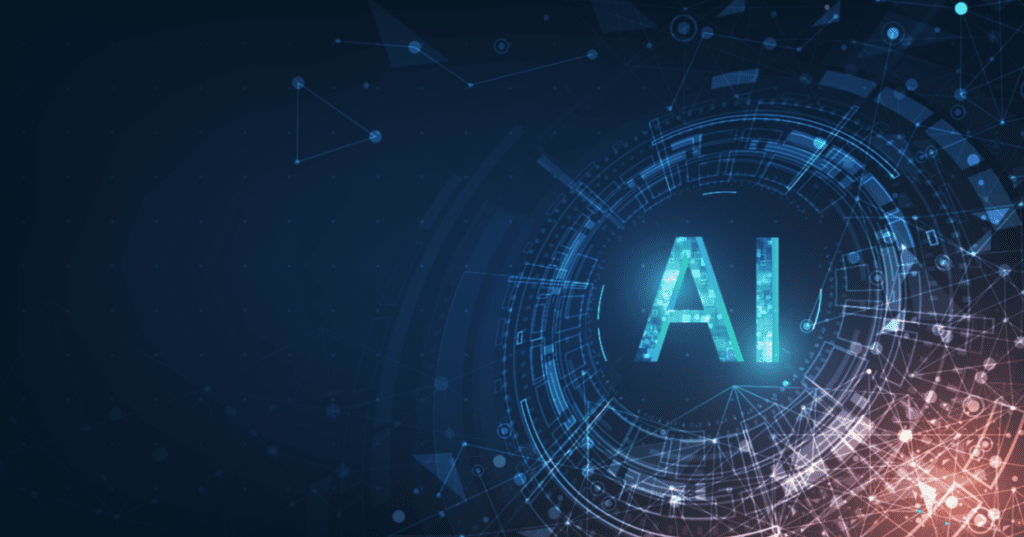
Unveiling the Synergy: Applications of Machine Learning and Artificial Intelligence in Cybersecurity
The Introduction
Machine learning and artificial intelligence were introduced to help cybersecurity professionals enhance the detection and prevention of cyber threats. The use of machine learning in cybersecurity can be traced back to the early 2000s when researchers began exploring its potential for identifying patterns in network traffic and detecting anomalies that could indicate malicious activity. Artificial intelligence, on the other hand, has been applied to cybersecurity for tasks such as automated threat detection, response, and decision-making.
The purpose of integrating machine learning and artificial intelligence into cybersecurity is to improve the accuracy and efficiency of threat detection and response for cybersecurity teams, as well as to enable proactive defense mechanisms against evolving cyber threats. Both of these technologies are powerful tools that will allow security systems to adapt and learn from new data, helping to stay ahead of sophisticated cyber attacks.
Machine Learning in Cybersecurity: Empowering Proactive Threat Detection
Machine learning has become a cornerstone of modern cybersecurity, revolutionizing threat detection, anomaly identification, and predictive analytics. Within cybersecurity, machine learning algorithms are leveraged to analyze vast volumes of security data, including network traffic, logs, and user behavior, to discern patterns indicative of potential threats. By autonomously learning from historical data and adapting to evolving threat landscapes, machine learning empowers cybersecurity systems to proactively detect and mitigate security threats, including malware, insider threats, and abnormal activities, with heightened precision and speed.
Artificial Intelligence in Cybersecurity: Orchestrating Intelligent Defense Mechanisms
Artificial intelligence augments cybersecurity defenses by orchestrating intelligent defense mechanisms that transcend the capabilities of traditional rule-based systems. Within cybersecurity, artificial intelligence is harnessed to minimize false positives, automate incident response, contextualize security events, and facilitate adaptive decision-making. By integrating natural language processing and knowledge representation, artificial intelligence enables cybersecurity systems to comprehend and respond to complex security events, minimizing repetitive tasks and enhancing the agility and efficacy of cyber defenses.
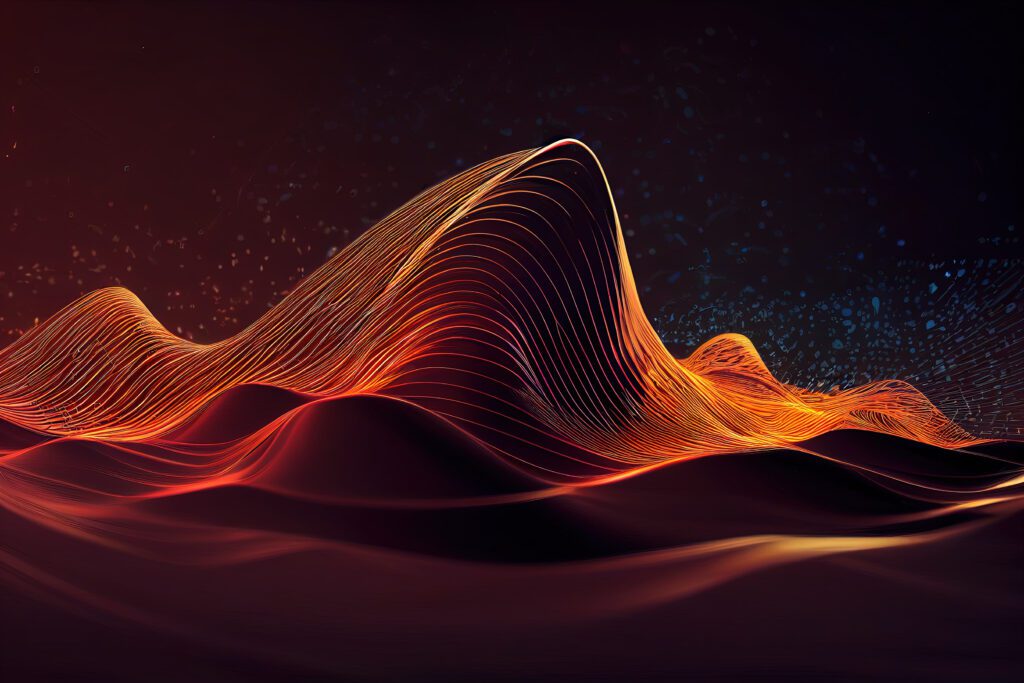
Convergence and Complementarity: Harnessing the Power of Both Technologies in Cybersecurity
Synergistic Integration: Uniting Machine Learning and Artificial Intelligence
Although machine learning and artificial intelligence are different approaches, when combined in cybersecurity, they work together to strengthen cyber defenses in a mutually beneficial way. Machine learning’s strength in discerning patterns and anomalies harmonizes with artificial intelligence’s capacity for intelligent decision-making and contextual understanding, culminating in a unified defense framework adept at detecting, contextualizing, and mitigating various cyber threats.
Adaptive Resilience: Leveraging Machine Learning and Artificial Intelligence Together
By combining machine learning’s ability to learn and adapt with artificial intelligence’s cognitive reasoning, cybersecurity systems can become more adaptable and robust. They can continuously learn, adapt, and respond to new cyber threats. This combination enables cybersecurity systems to proactively detect new attacks, identify subtle signs of compromise, and coordinate intelligent responses to security incidents. This makes organizations more resilient against the ever-changing and complex threat environment.
The Future of Cybersecurity: Advancing Cybersecurity Through Machine Learning and Artificial Intelligence
As the cybersecurity landscape continues to evolve, the integration of machine learning and artificial intelligence will continue to work together and grow to strengthen cybersecurity by further enhancing their capabilities in several ways by:
- Analyzing vast amounts of data to identify patterns and anomalies that could indicate potential cybersecurity threats will improve the accuracy and speed of threat detection. This will enable security systems to detect and respond to threats in real-time.
- Focusing on predictive analytics to uncover suspicious activities to anticipate and prevent cyber attacks before they occur. By analyzing historical data and identifying trends, machine learning, and artificial intelligence can help organizations proactively fortify their defenses against emerging threats.
- Advancing automated incident response and decision-making processes will enable security systems to respond to security incidents autonomously, minimizing the impact of attacks and reducing the burden on human security teams.
- Continuing to evolve in adapting to new and evolving threats. Machine learning and artificial intelligence will be trained on the latest threat intelligence and will continuously learn from new data to stay ahead of cybercriminals.
The convergence of these technologies holds the promise of enabling autonomous threat detection, adaptive defense mechanisms, and anticipatory risk mitigation, empowering security analysts to navigate the complexities of modern cyber threats with heightened efficacy and agility.
Distinguishing Between Machine Learning and Artificial Intelligence
Distinguishing between machine learning and true artificial intelligence (AI) in cybersecurity can be challenging. Still, there are some key indicators that can help a cybersecurity professional spot the difference:
Understanding of Context: Machine learning systems typically focus on learning from data and making predictions or decisions based on that data. True AI, on the other hand, can understand and reason about complex contexts, make decisions in ambiguous situations, and exhibit a deeper level of understanding.
Adaptability: Machine learning systems are often designed to excel at specific tasks based on training data, while true AI can adapt and learn across a wide range of tasks and domains, often without explicit programming.
Autonomy: True AI systems can exhibit more autonomy, making decisions and taking actions without human intervention, whereas machine learning systems often require human input for training and decision-making.
Creativity and Problem-Solving: True AI can demonstrate creativity and problem-solving abilities in novel situations, while machine learning systems are generally limited to the patterns and knowledge present in their training data.
Natural Language Understanding: True AI can understand and generate natural language in a way that goes beyond simple pattern recognition, while machine learning systems may be more limited in their language capabilities.
Understanding the capabilities and limitations of the technologies deployed within a security operations center will help cybersecurity experts better defend against adversarial attacks.
Advancing Threat Detection with Third-wave AI
Third-wave artificial intelligence, also known as “AI 3.0,” represents the latest evolution in artificial intelligence, characterized by its focus on contextual adaptation, explainability, and human-AI collaboration. Unlike its predecessors, Third-wave AI is designed to comprehend and adapt to complex real-world scenarios, provide transparent rationale for its decisions, and collaborate seamlessly with human operators. This paradigm shift in AI has profound implications for novel attack and threat detection, as it enables AI systems to contextualize security events, discern subtle indicators of compromise, and collaborate effectively with security teams to mitigate emerging threats.
MixMode: The Leader Advanced AI
MixMode is at the forefront of using third-wave artificial intelligence and is positioned to excel in novel attack and threat detection for several key reasons:
Contextual Adaptation: MixMode’s AI is adept at contextual adaptation, enabling it to comprehend the nuances of evolving cyber threats and discern abnormal behaviors within the broader context of an organization’s network environment. This contextual understanding empowers MixMode’s AI to identify novel attack vectors and emerging threats that may elude traditional security measures.
Explainable AI: MixMode’s AI is designed to provide transparent and explainable rationale for its threat detection decisions, enabling security teams to understand the underlying factors contributing to identifying potential threats. This transparency fosters trust and collaboration between the AI system and human operators, enhancing the overall efficacy of threat detection and response.
Human-AI Collaboration: MixMode’s AI is engineered to collaborate seamlessly with security teams, leveraging human expertise and AI capabilities synergistically. By facilitating effective collaboration between AI and human operators, MixMode empowers organizations to harness the collective intelligence of both entities, thereby enhancing the agility and precision of threat detection and response.
Adaptive Learning: MixMode’s AI continuously learns and adapts to evolving threat landscapes, enabling it to identify novel attack patterns and emerging threats proactively. This adaptive learning capability positions MixMode as a frontrunner in threat detection, as it ensures that the AI system remains effective in mitigating previously unknown vulnerabilities and sophisticated adversarial attacks.
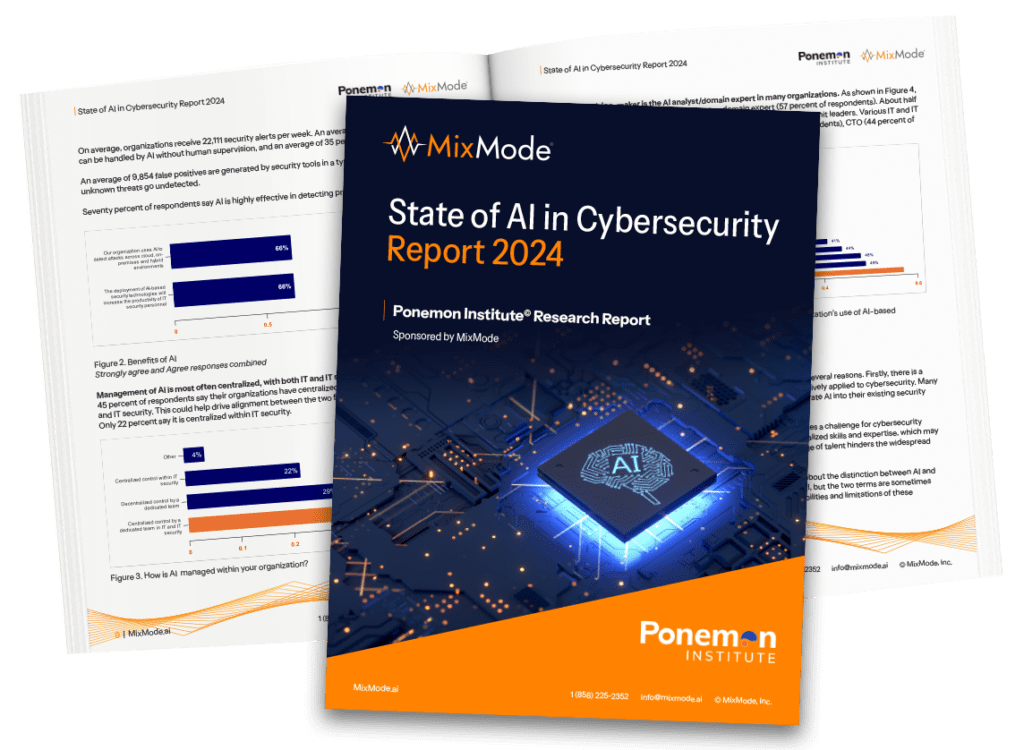
The distinctions between machine learning and artificial intelligence within cybersecurity are pivotal, as they underscore the diverse methodologies and applications underpinning these technologies. While machine learning excels in data-driven learning and proactive threat detection, artificial intelligence encompasses a broader spectrum of cognitive capabilities and intelligent decision-making. However, it is their convergence and complementarity that herald a new era of cyber resilience, empowering organizations to fortify their defenses against the ever-evolving cyber threat landscape.
MixMode’s pioneering utilization of Third-wave artificial intelligence for threat detection sets it apart. MixMode empowers organizations to fortify their defenses against novel attacks and emerging threats with unparalleled efficacy and agility by harnessing the contextual adaptation, explainability, human-AI collaboration, and adaptive learning capabilities of Third-wave AI.
Download MixMode’s inaugural State of Cybersecurity 2024 report to learn how organizations are harnessing AI’s power, or contact us to learn how MixMode’s AI can help bolster your defenses.
Other MixMode Articles You Might Like
AI and Cybersecurity: A Rob Burgundy Investigation
Chat GPT and Nation-State Attackers: A New Era of AI-generated Attacks
City of Dallas Selects the MixMode Platform to Fortify Its Critical Infrastructure
The Current State of SOC Operations Shows The Escalating Need for AI in Cybersecurity
MixMode Releases the First-Ever State of AI in Cybersecurity Report 2024